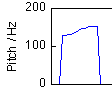
SAcC - Subband autocorrelation classification pitch tracker
SAcC is a (compiled) Matlab script that performs noise- robust pitch tracking by classifying the autocorrelations of a set of subbands using an MLP neural network. It has good resistance to noise, and is highly resistant to octave errors. You can read about it in our Interspeech 2012 paper, Noise Robust Pitch Tracking by Subband Autocorrelation Classification.
Contents
Example Usage
The Matlab script can be run from the Matlab prompt, or using the included Unix shell wrapper, run_SAcC.sh:
Run it over our demo files
% (1) the previous default sr=16k,bpo=16,sb=48,kdim=10 trained on RATS % SAcC files.list conf/rats_sr16k_bpo16_sb48_k10.config % (2) the faster new config sr=8k,bpo=6,sb=24,kdim=10 trained on RATS SAcC files.list conf/rats_sr8k_bpo6_sb24_k10.config % (3) the faster new config sr=8k,bpo=6,sb=24,kdim=10 trained on Keele % SAcC files.list conf/keele_sr8k_bpo6_sb24_k10.config % (4) Babel example with Babelnet config sr=8k,bpo=6,sb=24,kdim=10 % SAcC files_b.list conf/Babelnet_sr8k_bpo6_sb24_k10.config % (5) Babel example with RATS config sr=8k,bpo=6,sb=24,kdim=10 % SAcC files_b.list conf/rats_sr8k_bpo6_sb24_k10.config % (6) Babel example with Keele config sr=8k,bpo=6,sb=24,kdim=10 % SAcC files_b.list conf/keele_sr8k_bpo6_sb24_k10.config
Wrote ASCII-format out/rl001.SAcC.pitch Wrote ASCII-format out/rl002.SAcC.pitch Wrote ASCII-format out/sb001.SAcC.pitch Wrote ASCII-format out/sb002.SAcC.pitch
Load one of the example files and plot its pitch track
% (a) for files.list afn = 'audio/rl001.wav'; pfn = 'out/rl001.SAcC.pitch'; % (b) for files_b.list % afn = 'audio/BP_104.sph'; % pfn = 'out/BP_104.SAcC.pitch'; [d,sr] = audioread(afn); figure, subplot(311) specgram(d,256,sr); % Now load the pitch track file written by SAcC pt = textread(pfn); timestep = 0.010; subplot(312) plot(timestep*pt(:,2),pt(:,3)); ylabel('Pitch / Hz') % Also plot the voicing probability subplot(313) plot(timestep*pt(:,2),pt(:,4)); ylabel('P(voiced)')
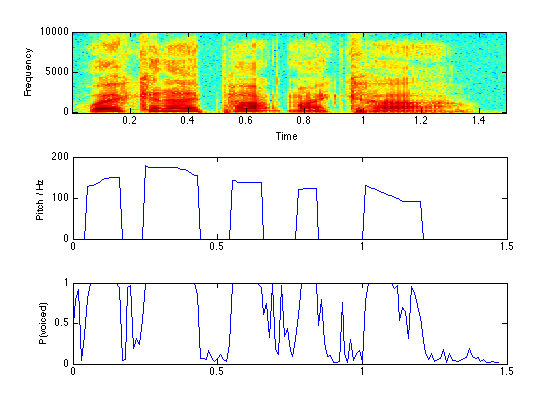
Training a new classifier
We now provide functions to support the training of new SAcC classifiers from training sets consisting of audio and ground-truth pitch tracks, or possibly consensus pseudo-ground truth as produced by the pt_mkPseudoGt package. This routine also relies on the pt_read.m function from that package, so it needs to be installed in a sibling directory.
Note: to work, this routine requires working binaries for pfile_create, qnnorm, and qnstrn in the path. These are part of the icsi-scenic-tools package.
% Train a new pitch tracker pitchdir = '../../data/pitch/keele'; idlist = textread(fullfile(pitchdir, 'idlist.txt'), '%s'); audiodir = fullfile(pitchdir, 'wav'); audioext = '.wav'; gtdir = fullfile(pitchdir, 'ptk', 'gt'); gtext = '-gt.txt'; name = 'keeleclean'; train_SAcC(idlist, audiodir, audioext, gtdir, gtext, name); % We can now run the newly-trained pitch tracker, using the new % config file confname = [name,'-config.txt']; P = config_read_srs(confname); [pfreq,lobs,pvx,times] = SAcC_main(afn, P); % Overplot on previous example subplot(312) hold on; plot(times, pfreq, '--r'); legend('default','retrained'); subplot(313) hold on; plot(times, pvx, '--r');
*** train_SAcC v1.74 of 20140123 *** Processing ../../data/pitch/keele/wav/f1nw0000.wav ... Processing ../../data/pitch/keele/wav/f2nw0000.wav ... Processing ../../data/pitch/keele/wav/f3nw0000.wav ... Processing ../../data/pitch/keele/wav/f4nw0000.wav ... Processing ../../data/pitch/keele/wav/f5nw0000.wav ... Processing ../../data/pitch/keele/wav/m1nw0000.wav ... Processing ../../data/pitch/keele/wav/m2nw0000.wav ... Processing ../../data/pitch/keele/wav/m3nw0000.wav ... Processing ../../data/pitch/keele/wav/m4nw0000.wav ... Processing ../../data/pitch/keele/wav/m5nw0000.wav ... pfile_create -i /private/tmp/tp1ff02632_962d_4b56_a04f_da1a3d25b4a0.txt -f 240 -o keeleclean-sbac.pf Did not delete /private/tmp/tp1ff02632_962d_4b56_a04f_da1a3d25b4a0.txt qnnorm norm_ftrfile=keeleclean-sbac.pf output_normfile=keeleclean-sbac.norms qnnorm was invoked with the following argument values (some of which may be defaults): norm_ftrfile=keeleclean-sbac.pf \ ftr_format=pfile \ ftr_width=0 \ delta_order=0 \ delta_win=9 \ output_normfile=keeleclean-sbac.norms \ first_sent=0 \ num_sents=2147483647 \ debug=0 \ verbose=true \ Program version: qnnorm v0_98. Host: dhcp-65-68.ee.columbia.edu Program start: Tue Feb 4 14:05:34 2014 Opening feature file... File contains 240 feature(s). Normalizing features. Norms file written: keeleclean-sbac.norms Program stop: Tue Feb 4 14:05:34 2014 Processing ../../data/pitch/keele/ptk/gt/f1nw0000-gt.txt ... Processing ../../data/pitch/keele/ptk/gt/f2nw0000-gt.txt ... Processing ../../data/pitch/keele/ptk/gt/f3nw0000-gt.txt ... Processing ../../data/pitch/keele/ptk/gt/f4nw0000-gt.txt ... Processing ../../data/pitch/keele/ptk/gt/f5nw0000-gt.txt ... Processing ../../data/pitch/keele/ptk/gt/m1nw0000-gt.txt ... Processing ../../data/pitch/keele/ptk/gt/m2nw0000-gt.txt ... Processing ../../data/pitch/keele/ptk/gt/m3nw0000-gt.txt ... Processing ../../data/pitch/keele/ptk/gt/m4nw0000-gt.txt ... Processing ../../data/pitch/keele/ptk/gt/m5nw0000-gt.txt ... pfile_create -i /private/tmp/tpd9f51cf9_9284_42d6_9bf8_c3cb7ada7cdb.txt -l 1 -o keeleclean-ptch67.pf train_mkMLP: qnstrn ftr1_file=keeleclean-sbac.pf hardtarget_file=keeleclean-ptch67.pf hardtarget_format=pfile ftr1_norm_file=keeleclean-sbac.norms ftr1_ftr_start=0 ftr1_ftr_count=240 window_extent=1 mlp3_input_size=240 mlp3_hidden_size=100 mlp3_output_size=68 train_sent_range=0:7 train_cache_frames=100000 cv_sent_range=8:9 learnrate_vals=0.008 ftr1_window_len=1 ftr1_window_offset=0 ftr1_delta_order=0 ftr1_delta_win=1 out_weight_file=keeleclean-sbac-ptch67-h100.wgt hardtarget_window_offset=0 log_file=keeleclean.log log_weight_file=keeleclean.chklog ckpt_weight_file=keeleclean.chk hardtarget_lastlab_reject=true Config params file written to keeleclean-config.txt
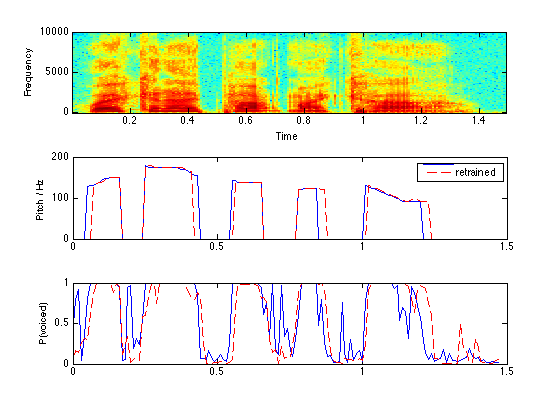
Installation
This package has been compiled for several targets using the Matlab compiler. You will also need to download and install the Matlab Compiler Runtime (MCR) Installer. Please see the table below:
Architecture | Compiled package | MCR Installer |
---|---|---|
64 bit Linux | SAcC_GLNXA64.zip SAcCsri_GLNXA64.zip train_SAcC_GLNXA64.zip |
Linux 64 bit MCR Installer |
64 bit MacOS | SAcC_MACI64.zip SAcCsri_MACI64.zip train_SAcC_MACI64.zip |
MACI64 MCR Installer |
You'll still need to download the source package below to get the parameter files.
The original Matlab code used to build this compiled target is available at http://labrosa.ee.columbia.edu/projects/SAcC/
All sources and the parameter files are in the package SAcC-v1.74.zip.
Feel free to contact me with any problems.
Alternative Classifiers
The package above includes four different classifiers, corresponding to the four different config files referenced in the examples. They differ in the sampling rate and structure of the subband filterbank (audio files are transparently resampled to the appropriate sampling rate, so this is a free choice), and in the data used to train the pitch classifier. The "keele" config is trained on the well-known Keele pitch database, with added pink noise between 0 and 20dB SNR. The "rats" configs are trained on high-noise radio channel data (from the RATS project). This works well on high-noise data, but tends to make voicing false-detections on more conventional low-noise data (such as telephone speech). For those applications, we came up with the "Babel" config which has been trained on a mix of high noise RATS data, then a few epochs with the cleaner Keele data. This is probably the best option for general applications.
Python Port
The core feature calculation and neural network pitch classifier has been ported to Python as part of the calc_sbpca package (see SAcC_list.py) It uses the same config files as this Matlab code. The Python code does not, however, include the functionality provided by train_SAcC to create new classifiers - you still need to use this Matlab code for that.
Changelog
% 2014-01-23 v1.74 - When I came back to this, train_SAcC above % wasn't working ?! Problem was in the format % of data returned by pt_read, which returns % rows but the code in train_mkLabFile was % expecting a column; fixed it, and fixed % freq2pitchix to quantize arbitrary matrices. % No changes to core SAcC, but version bumped % for consistency. % % 2013-03-14 v1.73 - added start_utt to config, to allow arbitrary % utterance numbering for write_rownum mode. % Also, incr_utt makes utterance number increment % for each utterance in write_rownum mode. % - includes train_make_ftr_file.sh to show how to % make a feature file for train_SAcC externally % (so it can be parallelized - see target % testtrnextftr in Makefile). % - extended last frame in PEM segments to % complete the final window (e.g., an extra 15 ms). % - slight change to ascii output format (now 5 % s.f. instead of 6 d.p.) % - random number generator (for dither) is now % reset at the start of each utterance to give % reproducible results. % % 2013-02-27 v1.72 - modified train_SAcC so that 7th argument, the % number of hidden layer units, can be specified % on the command line of the compiled binary. % % 2013-02-21 v1.71 - added a new binary output, train_SAcC, which % takes the same arguments as the train_SAcC % matlab function above. % % 2013-02-07 v1.7 - added train_SAcC to train a new net based on % audio files and corresponding ground-truth. % Also added write_rownum (default 1) and % write_time (default 0) flags so SAcC can write % pitch track files in the same format used by % the ptrack package. % % 2012-11-01 v1.6 - supports PEM files, specifying ranges of audio % file to process, as optional 3rd element in % each line of files.list file. % % 2012-09-26 v1.51 - config_default.m no longer tries to find % config files in $srcdir, but just defaults to % CWD. % % 2012-09-24 v1.5 - removed use of onCleanup in readmlpwts.m % - added options for write_posteriors, write_sbac, % and write_sbpca for different output data types. % Actual output is concatenation of all those % selected; new ones default to unselected. % Also added mat_out option to write output as a % MAT file with the features in a variable called % 'ftrs'. % % 2012-08-15 v1.41 Added write_pitch and write_pvx to config to % control which feature columns are saved. % % 2012-08-03 v1.4 Added support for lower sampling rates and % subband densities, allowing much faster instances. % Distribution now comes with 4 different configs; % the three new ones are 6-7x faster than v1.3. % % 2012-06-24 v1.3 Further intensive optimization of autocorrelation % (autocorr.c) for roughly 30% speedup. % % 2012-06-14 v1.2 Fixed segv bug where autoco ran off end of array % - some speedup to autocorrelation % - added SAcCsri, which uses SRI-format "metadb" config files. % % 2012-05-01 v1.1 Does not attept to plot, handles .sph file, % makefile setup. % % 2012-05-01 v1.0 Initial release %
Acknowledgment
This work was supported by DARPA under the RATS program via a subcontract from the SRI-led team SCENIC. My work was on behalf of ICSI.
$Header: /u/drspeech/data/RATS/code/SAcC/RCS/demo_SAcC.m,v 1.2 2012/08/03 16:50:51 dpwe Exp dpwe $